Forecasting household electricity loads
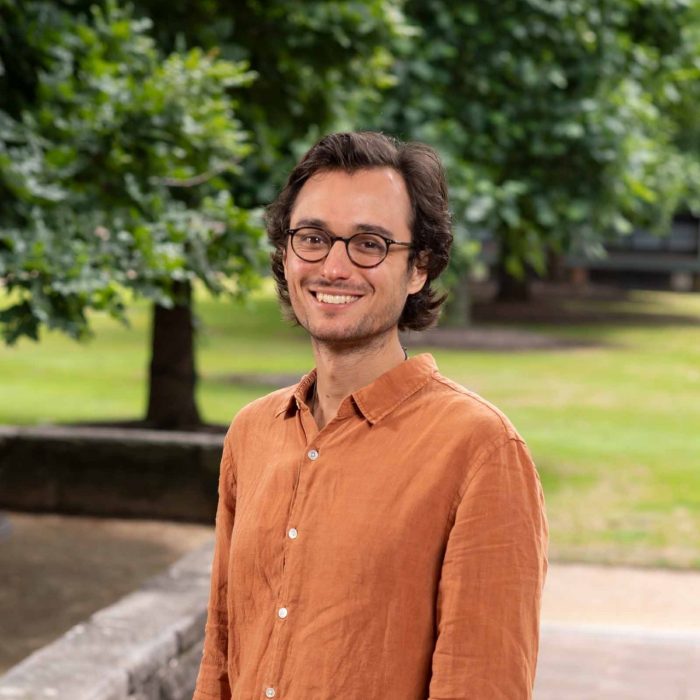
Forecasting household electricity loads with machine learning
The School of Photovoltaic and Renewable Energy Engineering (SPREE) worked across UNSW with the Centre for Low Carbon Living on this innovative data project with industry partner, Solar Analytics. Understanding household electricity demand and consumption habits is critical for the development of smart solar technologies that can both reduce carbon emissions (household electricity use generating a quarter of all energy produced) and the cost of electricity, with Australians paying some of the highest electricity bills in the world.
Smart home energy management systems are slowly finding their place in our homes and offices, where they can optimize the operation of appliances and help utilise solar power and batteries effectively, ultimately transforming them into energy-smart dwellings. In order to achieve this, it is important to improve our understanding of household consumption behavior by using smart algorithms and methods.
The project developed a novel hybrid statistical-machine learning model to process real-world data from thousands of Australian households as provided by Solar Analytics in order to accurately forecast the habitual usage behaviors of home energy consumers, focusing on the heating of electric hot water systems via utilizing excess solar generation. The results have shown that the information gained through the developed modelling can expand the capabilities of home energy management systems and help to improve the potential savings and reduce the associated emissions.
Baran Yildiz, PhD candidate on the project said of the experience: “It was highly valuable to collaborate with an industry partner during my PhD,” he explained. “Receiving regular feedback and having ongoing discussions with industry experts outside academia helped me apply the theoretical academic knowledge to pragmatic real-world problems.”