Epidemiology and biostatistics
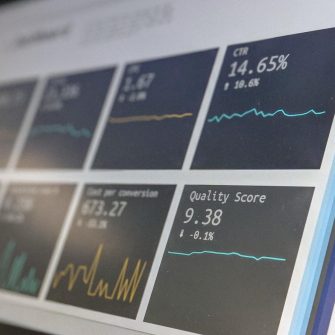
Big data for epidemiology and biostatistics
At the Centre for Big Data Research in Health (CBDRH), we define our research questions alongside clinicians and policy makers. We design epidemiologically rigorous studies using linked administrative data and we secure the proper ethical approvals to link datasets.
Our researchers manage data by developing governance and documentation for complex research data assets. We're managing data access, technologies and statistical disclosure control for sensitive health and personal data. We also clean and wrangle data to prepare research-ready datasets from multiple linked data sources.
The statistical analyses we perform engage modern statistical approaches for addressing different research aims. This includes descriptive analysis, prediction and estimating causal effects. Our methods for analysing data from different study designs include observational data, cohort studies and clinical trials. The methods for analysing data with complex structure include longitudinal data, clustered data and time series data. Quasi-experimental designs for estimating the effects of programs and interventions use observational data. Methods to account for common biases in research data include, measurement error, selection bias and missing data bias. The expertise we use for software and packages for analysing health data include R, Python, SAS and Stata.
We disseminate our results by developing data infographics, visualisations and interactive dashboards to bring our results to life. We then communicate these results across a range of modes and to diverse audiences—from publishing in academic journals to presenting to clinicians and policymakers and in writing for lay audiences
We also provide regular, expert commentary to media outlets on health, data and research issues.