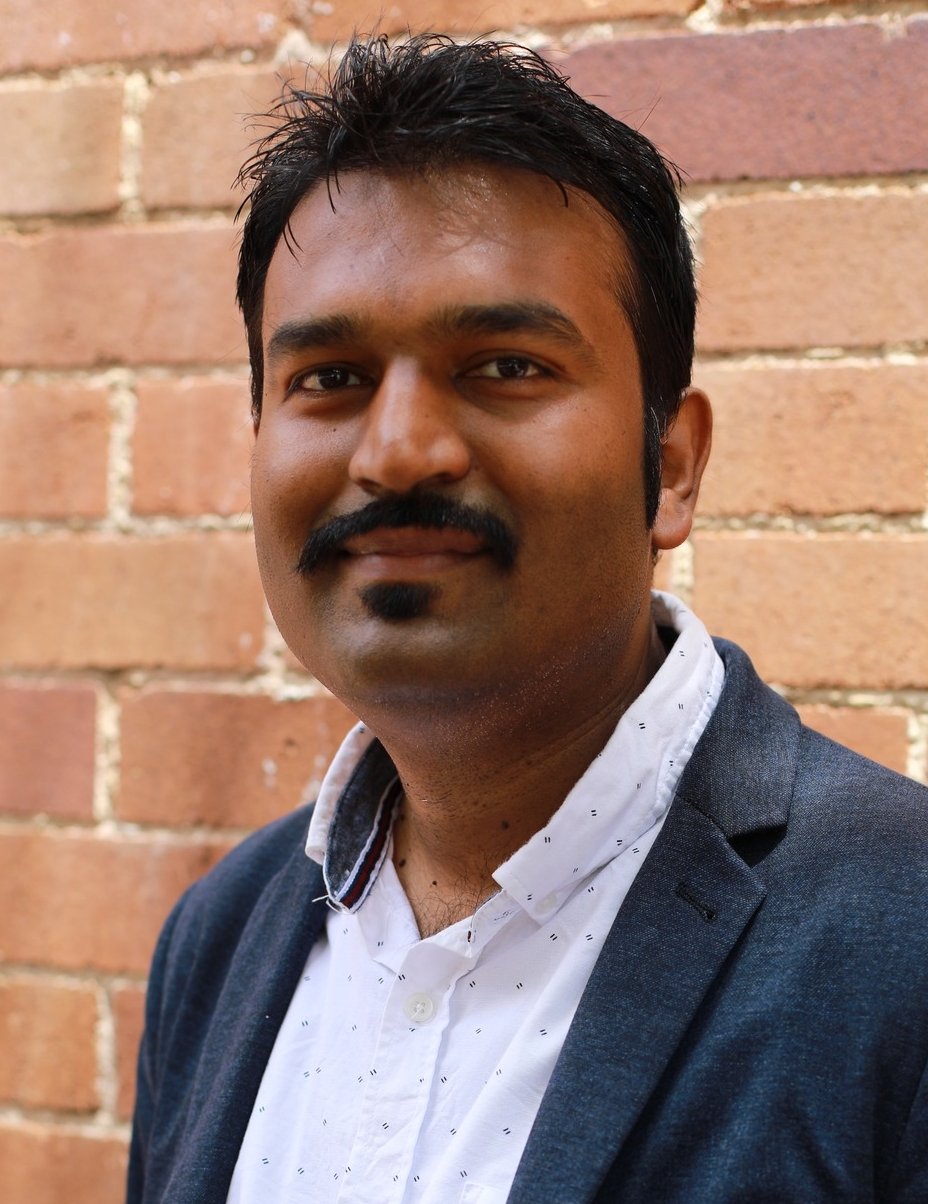
Dr Rohitash Chandra
- PhD in Artificial Intelligence, Victoria University of Wellington (2012)
- MSc. in Artificial Intelligence, University of Fiji (2008)
- BSc. in Computer Science and Engineering Technology, University of the South Pacific (2006)
Dr Rohitash Chandra is a Senior Lecturer in Data Science at the UNSW School of Mathematics and Statistics. He leads a program of research encircling methodologies and applications of artificial intelligence. The methodologies include Bayesian deep learning, neuroevolution, ensemble learning, and data augmentation. The applications include climate extremes, geoscientific models, mineral exploration, biomedicine, and COVID-19 where the focus has been drug selection, infection forecasting, and social media-based language modelling. Dr Chandra has also pioneered the area of language models for studying ancient religious-philosophical texts. Dr Chandra used machine learning and remote sensing for mineral exploration and is currently focusing on critical metals. He has been using machine learning for climate extremes and currently developing a model for decadal forecasts of high-category cyclones.
Dr Chandra is the Data Theme Lead of the Australian Research Council (ARC ITTC) Training Centre for Data Analytics in Minerals and Resources (2020-2025). Dr Chandra has been one of the Chief Investigators of the NHMRC Medical Research Future Fund (2021-2022) project on the use of machine learning for COVID-19 drug repurposing. Apart from science, Dr Chandra takes a lot of interest in literature and humanities and has edited and published poetry collections. Dr Chandra is a strong advocate of human rights and diversity and is a UNSW Cultural Diversity Champion (2021-2023).
Prior to joining UNSW, Dr Chandra held a Chancellor's Research Fellowship at the University of Sydney (2017 - 2019). Prior to this, he has taken roles as a Research Fellow in Machine Learning at Rolls Royce @Corp Lab, Nanyang Technological University, Singapore; Postdoctoral Research Fellow in Bioinformatics at Victoria University of Wellington (January to June 2012), and Lecturer in Computing Science at the University of the South Pacific (2013- 2015). Dr. Chandra is originally from Fiji (Nausori) and has a Girmit Indian heritage.
Dr Chandra is on the Editorial Board for Geoscientific Model Development published by the European Geoscience Union (EGU). He also served as an Associate Editor for IEEE Transactions on Neural Networks and Learning Systems, and Neurocomputing (2021-2022). Dr. Chandra is a Senior Member of IEEE and an Associate Fellow of the British Higher Education Academy (HEA). Dr Chandra is the founding director of the Transitional Artificial Intelligence Research Group (t-AI) based at UNSW Sydney. Since 2021, Dr Chandra has been featured in the Stanford's list of World Top 2% scientists. His research publication culture demonstrating leadership is given by the first, last, and corresponding author which makes more than 90% of his publications. Furthermore, 85% journal articles appear in Q1 journals (Citescore and SJR).
- Publications
- Media
- Grants
- Awards
- Research Activities
- Engagement
- Teaching and Supervision
- S. Vasan and R. Chandra et al., "The sySTEMs initiative: systems biology-augmented, stem cell-derived, multi-tissue panel for rapid screening of approved drugs as potential COVID-19 treatments," NHMRC - Medical Research Future Fund (MRFF), July 2021- June 2022 ($1,000,000),
- S. Cripps, R. Chandra, et al., "ARC training centre in data analytics for resources and environments (ARC ITTC DARE)," 2020 - 2024: https://darecentre.org.au/ ($4,000,000 from ARC and $6,500,000 in-kind support from industry)
- R. Chandra, Sydney Fellowship Awards, DVC Research, University of Sydney, 2017 -2019
- D. Muller, R. Chandra, et al., Understanding the deep carbon cycle from icehouse to greenhouse climates, Sydney Research Excellence Initiative (SREI), DVC Research, University of Sydney, 2017 - 2018 ($300,000)
- Doctoral Completion Award, Victoria University of Wellington (2012)
- Sydney Fellowship Award, University of Sydney (2017-2019)
- UNSW Science Silverstar Award 2022, Faculty of Science, UNSW
Research Themes
We developed a transdisciplinary program of research encircling methodologies and applications of data science. The methodologies include Bayesian deep learning, neuroevolution, ensemble machine learning, and data augmentation. The applications include climate extremes, geoscientific models, mineral exploration, medical diagnosis and bioinformatics, and COVID-19 drug re-purposing, infection forecasting, and social media with language models. Our key strength is in the development of novel deep-learning software frameworks with a focus on uncertainty quantification in decision-making. We pioneered the area of language models for studying ancient religious-philosophical texts.
Machine Learning
- Machine learning and deep learning: We developed novel neural network learning algorithms using neuroevolution with motivations from transfer learning and multi-task learning to a wide range of problems that include multi-step ahead and dynamic time series prediction (Chandra et al., 2017; Chandra et al., 2018) and modular pattern recognition for dynamic environments (Chandra and Cripps, 2018) with the goal of modular deep learning. The challenge has been problems that have missing information, noise and inconsistencies in the organisation of data and these are major research directions for future studies. In order to address class imbalance problems and limited training data, we used generative adversarial networks (GANs) with machine learning models for data augmentation (Sharma et al., 2022). Our current focus is on extending the method to extreme-value forecasting, and also combining it with Bayesian inference for uncertainty quantification in predictions via the posterior distribution.
- Bayesian deep learning: We developed novel algorithms for Bayesian neural networks that feature parallel tempering MCMC and parallel computing in order to address computational challenges (Chandra et al., 2019}. We later extended this for deep learning models that feature millions of parameters that were “believed to be unachievable” in the MCMC sampling community. We developed Bayesian autoencoders using MCMC (Chandra et al., 2022) and Bayesian Graph convolutional neural networks (Chandra et al., 2022). Taking into account the benefits of evolutionary algorithms, we developed a framework that provides a synergy of multi-source transfer learning with Bayesian neural networks using MCMC (Kapoor et al., 2022). We plan to enhance the Bayesian deep learning models with data augmentation methods for multi-modal data fusion utilising a wide range of data streams. Furthermore, we plan to use a combination of variational inference and MCMC sampling methods to provide uncertainty quantification in the data and the model space.
Earth and Climate Sciences
- Remote sensing and machine learning: There is a huge potential for remote sensing when combined with emerging machine learning and deep learning methods. We demonstrated that the extraction of geological lineaments from satellite data via remote sensing and machine learning can be used for mineral exploration (Farahbakhsh et al.,2020). In collaboration with the EarthByte Group, we developed deep learning models for lithological mapping via remote sensing (Shirmard et al.,2022), and applied the same technology for the detection of alteration zones for mineral exploration. Currently, we are using variational autoencoders and remote sensing for the identification of lineaments with novel clustering methods. We are planning to use geochemical datasets with spatial machine learning and data augmentation methods for prospectively of critical metals in Australia. Furthermore, we are currently developing an open-source framework for land-cover mapping in Fiji where we plan not only to publish a paper but to release online digital maps that can help in environment restoration initiatives. We plan to extend these methods for space exploration projects with the study of the surfaces of the Moon and Mars using satellite data. We also plan to apply these methods to environmental protection and conservation problems, such as quantifying damages to reefs after high-category cyclones and monitoring invasive species (eg. African tulips in Fiji).
- Machine learning for the GBR: The Great Barrier Reef (GBR) is the world’s largest coral reef system which is vital for a healthy ecosystem in Australia and the Pacific Ocean. In collaboration with the Geocoastal Research Group (University of Sydney), we used remote sensing and clustering methods for reef community mapping from the One-Tree Island of the Great Barrier Reef (Barve et al., 2023). Our interest is in the study of the geological development of the GBR going back thousands of years in time. We developed an open-source software framework for reef modelling (Bayesreef) that provides insights into coral platform growth and demise through time (Pall et al, 2020) using the Py-Reef-Core model. We developed a machine learning framework for processing coral reef drill core data (Deo et al. 2023). Currently, we are using novel deep learning models for the study of organisms on the deep sea floor in collaboration with the University of Sydney and Imperial College London. In future, we are interested to use machine learning and Bayesian inference methods in connecting landscape evolution models with reef evolution models to have a better understanding of the development of the GBR.
- Climate extremes and environmental problems: The drastic effect of climate change is visible given extreme weather conditions such as tropical storms and cyclones. We used deep learning models for forecasting cyclone wind intensity and trajectory for South Pacific and South Indian Oceans (Chandra and Dayal, 2015; Deo and Chandra, 2016; Chandra et al.,2016 ). We also focused on the rapid intensification of cyclones (Chandra, 2017) and uncertainty quantification in predictions using Bayesian neural networks (Deo and Chandra, 2019). We developed variational deep learning models for cyclone trajectory prediction with uncertainty quantification (Kapoor et al., 2023). Currently, we are using deep learning for forecasting cyclone genesis in the coming decades given drastic changes in the climate via sea surface temperature data from the general circulation model (GCM) in collaboration with the Natural Hazards and Climate Risk group, Data 61. Furthermore, we used novel deep learning models for modelling Australian floods by taking into account precipitation and stream flow which led to an Honours thesis with potential publication. We are also combining hydrological models with deep learning models for modelling stream flow. We collaborated with UNSW Water Research Laboratory where we use deep learning models for groundwater modelling. Apart from climate extremes, we developed a framework that featured machine learning methods to predict precipitation that defines paleoclimate that spans up to millions of years in the past (Chandra et al., 2021). The data features a range of geological indicators including sedimentary deposits (coal, evaporates, glacial deposits). We addressed the challenges of missing values in the dataset and uncertainty quantification with Bayesian machine learning and developed paleo-maps of forests and vegetation that span 250 million years. In future work, we would like to use these maps and connect them with other paleoclimate studies.
- Bayesian geoscientific models: Bayesian inference has been a popular methodology for parameter estimation in geological and geophysical forward models, also known as geoscientific models. In collaboration with the EarthByte Group (University of Sydney), we developed Bayesian inference via MCMC framework for parameter estimation and uncertainty quantification for landscape evolution models (Bayeslands) to demonstrate landscape evolution in synthetic models that span thousands to million years demonstrating surface evolution based on different climate and environmental conditions (Chandra et al., 2019, Chandra et al., 2020). We envision that Bayeslands and Bayesreef will create a significant impact on the research community. We addressed the computational inefficiency of MCMC for large-scale problems by combining parallel computing features with a surrogate-assisted estimation of likelihood function that describes the plausibility of a model parameter value, given observed data (Chandra et al., 2020). We plan to use our MCMC sampling framework for estimating parameters in hydrological models combined with deep learning models.
Bioinformatics and Medicine
- Medical diagnosis and bioinformatics: In this area, the focus is on applying novel machine learning and deep learning models for medical diagnosis and bio-informatics that includes COVID-19. In NHMRC funded project, we developed an unsupervised machine learning framework for COVID-19 drug re-purposing (Bansal et al., 2023) where the goal was to down-select a small subset of drugs for COVID-19 clinical trials. We also used deep learning for COVID-19 infection forecasting in India (Chandra et al., 2022). Furthermore, we used deep learning models for respiratory rate prediction using bio-signals (Kumar et al., 2022). Currently, I am leading projects where we use deep learning models for skin cancer detection given imbalanced and limited training data. In future, we plan to use Bayesian deep learning for a wide range of medical diagnoses and health-related datasets where uncertainty quantification in model predictions plays a vital role in decision-making.
Media and Religion
- Language models for social media analyses: In this research direction, we used novel deep learning models to develop language models via social media to understand public behaviours in events such as COVID-19 (Chandra and Krishna, 2021). Currently, I am leading a project that reviews anti-vaccine tweets during COVID-19 with sentiment analysis. I led a related project that used sentiment analysis to model US 2020 Presidential elections (Chandra and Saini, 2021). Currently, we are working towards the analysis of political leaning (left vs right wing) in popular news media reporting using pre-trained deep learning-based language models. In the future, we plan to evaluate the right and left-wing biases of developed and emerging economies and study how it impacts political and economic development. We are currently developing models to study the emergence of Hinduphobia in India and around the world during COVID-19 via Twitter using sentiment analysis.
- Artificial intelligence for religion: There is an immense potential for data science methods and language models in areas of arts and humanities, particularly philosophy and religion. The Bhagavad Gita is a sacred Hindu philosophical text that is one of the most translated texts over the course of history. We used language models to analyse the sentiments uncovered with philosophical issues presented in the Bhagavad Gita and study the difference when comparing prominent translations (from Sanskrit to English) (Chandra and Kulkarni, 2022). We demonstrated that deep learning-based language models can be used to compare related texts, i.e. the Bhagavad Gita with Upanishads via topic modelling (Chandra and Ranjan, 2022). Currently, we are developing model for metaphor detection in the Bhagavad Gita and Sermon of the Mount of the Holy Bible. We are also using sentiment analysis for the comparison of translations of the Sermon of the Mount. In collaboration with Midam Charitable Trust in India, we evaluated Google Translate Sanskrit using Bhagavad Gita translations for comparison (Shukla et al., 2023). Currently, our focus is on large language models such as Chat-GPT for developing Vedanta-GPT. This model will be trained with Bhagavad Gita and Upanishads that will be released as an online discussion chat-bot for Hindu philosophy. We are also developing language models for humour detection in Hindu texts, and plan to use related language models for Buddhist texts. In future, we plan to use computer vision methods for the automatic archival of ancient sacred scripts in India in collaboration with the Oxford Centre for Hindu Studies.
Economics and Society
- Deep learning for forecasting future economic trends: We developed Bayesian neural networks that feature uncertainty quantification in forecasting future trends in the stock price of selected markets (Chandra and He, 2021). This led to a project that used recurrent neural networks for decadal economic forecasting focusing on country-wise GDP growth (Wang et al., 2023). Currently, we are developing novel deep learning models for the decadal cost of living outlook and variational deep learning for uncertainty quantification in stock price forecasting. We also used multimodal deep learning that incorporates text and numeral data streams with large language models for credit rating forecasting. In future, there is scope for multimodal data fusion for forecasting decadal economic trends taking into account, energy utilisation, climate change, migration and estimates of poverty elimination.
Available Research Projects
- Remote sensing for landscape detection on Mars (Honours/PhD) with Prof. Cedric John (Imperial College London)
- Detection of hazards from cyclones using remote sensing and deep learning (Masters/Honours) with Dr Ehsan Farahbakhsh (University of Sydney)
- Bayesian deep learning for hydrological models (Honours/PhD) with Prof. Willem Vervoort (University of Sydney)
- Explainable recurrent neural networks (Honours/PhD) with Prof. Christian Omlin (University of Agder, Norway)
- Language models for the analysis of philosophical traditions emerging from Hindu texts (Honours/PhD) with Dr Bjarne Wernicke-Olesen (Oxford Centre for Hindu Studies, Oxford University)
- Explainable artificial intelligence (Honours/PhD) with Prof. Giles Hooker (University of Pennsylvania, United States)
- Bayesian deep learning with incomplete information (Honours/PhD) with Prof. Scott Sisson (UNSW)
- Bayesian deep learning for crowd simulation and robot path prediction (Honours/PhD) with Dr William Zhi (Carnegie Mellon University, United States)
- Indoor path navigation for disabled persons in large buildings (Masters/Honours) with Dr William Zhi (Carnegie Mellon University, United States)
- Bayesian deep learning for robot path planning (Honours/PhD) with Prof. Bibhya Sharma (University of the South Pacific, Fiji)
- Data augmentation and deep learning (Honours/PhD) with Dr Anurag Sharma (University of the South Pacific, Fiji)
- Deep learning for medical diagnosis (Honours/PhD) with Dr Amit Krishan Kumar (Da Nang University, Vietnam)
- Decadal economic trends with deep learning (Honours/PhD) with Dr Rodney Beard (FFA, Solomon Islands) and Dr John Hawkins (Sydney)
- Sentiment analysis with deep learning during natural disasters and extreme events (Honours/Masters/PhD)
- Deep learning for protein function detection (Honours/PhD) with Prof. Alok Sharma (RIKEN, Japan)
- Plate tectonics for mineral prospecting with machine learning (Honours/PhD) with Prof. Dietmar Muller (University of Sydney)
- Machine learning for reef modelling and optimisation (Honours/PhD) with Prof. Jody Webster (University of Sydney)
Seminar Presentation
- R. Chandra, ``Natural language processing for philosophy of religion: Analysis of translations, sentiments and topics in religious texts", and Centre of Data Analytics and Cognition, LaTrobe University (August 2022), IIT Guwahati (November 2022), and University of the South Pacific (February 2023). Youtube
- R. Chandra, ``Unsupervised machine learning framework for discriminating major variants of concern during COVID-19", Lymphatic Medicine Laboratory, Monash Institute of Pharmaceutical Sciences, Monash University, August 2022. Youtube
- R. Chandra, ``Global COVID-19 Twitter dataset and language models for sentiment analysis and topic modelling", Melbourne University (August 2022), University of Tasmania (September 2022), and IIT Bombay (December 2022). Youtube
- R. Chandra and Ehsan Farahbakhsh, ``Machine learning for mineral exploration: a data odyssey", CSIRO Mineral Exploration, Perth (September 2022) and Fiji National University (February 2023). Youtube
- R. Chandra, ``Bayesian deep learning via MCMC sampling with application to robot path planning", Robotics and Automation Group, Queensland University of Technology, Brisbane, September 2022. Youtube
- R. Chandra, “Machine learning for paleo-geology and mineral exploration: A spatiotemporal odyssey”, ARC ITTC Data Analytics in Resources and Environments, December 2021. Youtube
- R. Chandra, “BERT-based language models for US Elections, COVID-19, and Bhagavad Gita”, UNSW Statistics Seminar Series, December 2021. Youtube
- R. Chandra, “Revisiting Bayesian deep learning with advancements in MCMC”, University of Auckland, Department of Statistics, April 2021. Youtube
- R. Chandra, “Unravelling Earth’s geological history with geoscientific models powered by artificial intelligence” University of the South Pacific, Public Seminar Series, September 2019. Youtube
- R. Chandra, ``Bayesian inference for Geoscientific models'', School of Computer Science, University of Wollongong, February 2019.
- R. Chandra, ``Bayesian inference for computationally expensive Earth evolution models'', School of Computer Science, University of Adelaide, October 2018. Youtube
- R. Chandra, ``Bayesian inference for modelling geo-coastal, basin and landscape evolution'', Basin Genesis Hub Workshop, The University of Sydney, February 2018.
- R. Chandra, `` Tackling climate change problems with machine learning '', EarthByte Group, School of Geosciences, The University of Sydney, July 2017. Youtube
- R. Chandra, ``Competitive neuroevolution with applications'', Seminar, School of Computing, Information and Mathematical Sciences, University of South Pacific, August 2015. Youtube
- R. Chandra, ``Open source software for education in Fiji'', Seminar, South Pacific Computer Society, University of South Pacific, Suva, Fiji, April 2013.
- R. Chandra, ``Chaotic time series prediction using recurrent networks", Seminar, Victoria University of Wellington, Wellington, New Zealand, August 2011.
My Research Supervision
PhD research students
- Arpit Kapoor, ''Bayesian deep learning for climate extremes and hydrological models", School of Mathematics and Statistics, UNSW Sydney, since September 2022 (Principal Supervisor with Dr Sahani Pathiraja, External supervisor- Prof. Lucy Marshall)
- Amuche Igenegbu, ''Explanable artificial intelligence for environment and climate problems", School of Mathematics and Statistics, UNSW Sydney, since February 2024 (Principal Supervisor with A/Prof. Pierre Lafaye de Micheaux)
- Saman Forouzandeh, "Recommender systems using graph-based deep learning'', School of Mathematics and Statistics, UNSW Sydney, since April 2023 (Joint Principal Supervisor with Dr Pavel Krivitsky)
- Ratneel Deo, ''Deep learning for understanding geo-coastal and reef development``, University of Sydney, from July 2021 (External Supervisor with Prof. Jody Webster and Dr Tristan Salles)
- Mahsa Tavakoli, "Synergy of language models and time series models for credit rate forecasting", Western University, Canada, since March 2022 (External supervisor with A/Prof. Cristian Roman)
Masters and Honours
- Xuechun Wang, "Evaluation of Google Translate for selected Chinese texts: sentiment and semantic analysis", Honours in Data Science, from T3 2023
- Chen Wang, "Sinophobia during COVID-19: Twitter ", Honours in Data Science, from T3 2023
- Tanay Panat, " Ease of living index by country", Masters in Data Science, T2 2024
Completions (PhD)
- Megan Nguyen, "Bayesian Inference for Complex Models", University of Sydney, September 2024 (External Supervisor with A/Prof. Minh-Ngoc Tran and Dr Tongliang Liu)
- Amit Kumar, "Multimodal approach for clustering risk level in pulmonary fibrosis patients using respiratory and EMG data", Beijing Institute of Technology, April 2023 (External Supervisor)
- Ehsan Farahbakhsh, “Developing a novel method for three-dimensional modelling of ore deposits by integrating data layers”, Amirkabir University of Technology, Tehran, December 2020 (External Supervisor)
Completions (Honours thesis)
- Shuhao Huang, "Explainable artificial intelligence for drought prediction in Australia", Honours in Computer Engineering, May 2024
- Albert Demskoy, "Bayesian model for high category cyclone forecasting using sea-surface temperature: four decades ahead", Honours in Data Science, December 2023
- Rahul Ahluwalia, "Data augmentation for extreme value forecasting using deep learning", Honours in Data Science, December 2023
- Sean Luo, "Evaluation of GANs with dimensional reduction approach", Honours in Data Science, May 2022 (Joint supervision with Dr Sahani Pathiraja)
- George Maksour, "Evaluation of deep reinforcement learning models for horse-race betting", Honours in Data Science, May 2022 (Joint supervision with Dr Sahani Pathiraja)
- Jim Ng, “ Conditional ensemble deep learning for modelling Australian climate extremes: streamflow and floods“, Honours thesis, UNSW Sydney, December 2022 (Primary Supervisor with A/Prof Willem Vervoot)
- Eric Chen, “Deep learning for modelling historic ground-water levels via stream-flow and precipitation data”, Honours thesis, UNSW Sydney, December 2022 (Primary Supervisor with A/Prof Martin Anderson)
- Royce Chen, "Pruning Bayesian neural networks with MCMC", Honours thesis, UNSW Sydney, December 2022 (Joint supervision with Dr Sahani Pathiraja)
- George Bai, "Bayesian neural ensemble learning with parallelized and tempered Langevin MCMC", Honours thesis, UNSW Sydney, December 2021 (Principal Supervisor)
- Jodie Pall, “ Bayesreef: Reef evolution using Bayesian inference”, Honours thesis, School of Geosciences, University of Sydney, December 2018 (Received University Medal) (Secondary Supervisor with Prof. Jody Webster and Dr Tristan Salles)
Completions (Masters by Research)
- Honghui Wang, "Deep learning for instant pedestrian path prediction", Masters by Research, 2024(Principal Supervisor with A/Prof Gustavo Batista and Dr William Zhi)
- Chaarvi Bansal, “Machine learning Framework for COVID-19 Drug Repurposing”, M.Sc. Biological Sciences, Birla Institute in Technology and Science Pilani and UNSW Sydney, 2022 (Principal Supervisor with Prof. P. R. Deepa )
- Julian Rodriguez, "Machine learning for spatial-temporal mineral prospecting using plate tectonic models, M.Phil, University of Sydney (External Supervisor with Prof. Dietmar Muller, 2019-2020)
- Ratneel Deo, “Neural network methodologies for cyclone wind intensity and path prediction”, Master of Science in Computing Science, University of the South Pacific, Suva, Fiji, December 2017 Download thesis from USP Library (Primary Supervisor - External Supervisor) (Nominated for Best Thesis - Gold Medal)
- Shonal Chaudhary, “Mobile Based Face Recognition for Visually Impaired Persons”, Master of Science in Computing Science, University of the South Pacific, Suva, Fiji, August 2015 Download thesis from USP Library (Primary Supervisor)
- Swaran Ravindra, “Health Information Systems in Fijian Hospitals”, Master of Science in Information Systems (Minor Thesis), University of the South Pacific, Suva, Fiji, 2015 Download thesis from USP Library (Primary Supervisor)
- Kavitesh Bali, “Competitive Island Cooperative Coevolution for Real Parameter Global Optimization”, Master of Science in Computing Science, University of the South Pacific, Suva, Fiji, September 2015 Download thesis from USP Library (Awarded PhD Scholarship at Nanyang Technological University - Singapore, 2016) (Awarded Gold Medal for Best MSc Thesis at USP) (Primary Supervisor)
- Ravneil Nand, “Competitive Island Cooperative Neuro-Evolution for Time Series Prediction”, Master of Science in Computing Science, University of the South Pacific, Suva, Fiji, January 2016 Download thesis from USP Library (Primary Supervisor)
- Shamina Hussein, “Multi-step ahead prediction using Recurrent Neural Networks”, Master of Science in Computing Science, University of the South Pacific, Suva, Fiji, 2015 Download thesis from USP Library (Primary Supervisor - External Supervisor)
- Shelvin Chand, “Multi-Objective Cooperative Neuro-Evolution for Chaotic Time Series Prediction”, Master of Science in Computing Science, University of the South Pacific, Suva, Fiji, August 2014 Download thesis from USP Library (Awarded PhD Scholarship at UNSW Australia, 2015) (Primary Supervisor)
Recent completions - Masters by Coursework (Minor Thesis)
- Yeshwanth Rayavarapu, "Comparison of GPT and Google Translate for translation of selected Indian languages", Masters in Data Science, May 2024, (Principal Supervisor)
- Ruoni Wen, "Remote sensing and deep learning for landcover classification in Fiji", Masters in Statistics, August 2023, (Principal Supervisor) , Co-supervisor Dr Ehsan Farahbakhsh)
- Alex Bradford, "Variatianal deep learning for stock price prediction", Masters in Statistics, August 2023, (Principal Supervisor)
- Mukuan Hsu, "Topic modelling for COVID-19 vaccine-related tweets", Masters in Computing Science, August 2023, (Principal Supervisor)
- Hamish Haggerty, “ Self supervised deep learning”, Masters in Statistics, May 2023, (Principal Supervisor)
- Tianyi Wang, Revisiting world economic outlook post-COVID-19 with deep learning", Master of Statistics, UNSW Sydney, December 2022, (Primary Supervisor)
- Yuhao Ke, “Machine learning for NBA”, Master of Statistics, School of Mathematics and Statistics, UNSW Sydney, December 2022 (Primary Supervisor)
- Mingyue Kang, "COVID-19 mutation over time", Master of Statistics, UNSW Sydney, May 2022 (Principal Supervisor in collaboration with Prof. Seshadri Vasan (CSIRO)
- Jiaxin Cathy Yu, "COVID-19 diagnosis study with big data", Master of Statistics, UNSW Sydney, May 2022 (Principal Supervisor in collaboration with Prof. Seshadri Vasan (CSIRO)
- Kelin Liu, "Clustering methods for vessel tracking with satellite data", Master of Statistics, UNSW Sydney, May 2022 (Principal Supervisor in collaboration with Dr Rodney Beard (FFA)
- Zhilin Wei, "Computer vision for aerial tracking of coastal plastic waste", Master of Statistics, UNSW Sydney, December 2021 (Principal Supervisor)
- Dizhou Feng, "Graph neural networks for spatiotemporal forecasting", Master of Statistics, UNSW Sydney, December 2021 (Principal Supervisor)
- Yueyang Zhang, "Gradient Boosting LSTM for reducing model uncertainty", Master of Statistics, School of Mathematics and Statistics, December 2021 (Principal Supervisor)
- Shaodong Lin, "World economic outlook post-COVID-19 with deep learning", Master of Statistics, School of Mathematics and Statistics, December 2021 (Principal Supervisor)
- Yixuan He, "Bayesian neural learning for financial prediction", Master of Financial Mathematics, UNSW Sydney, August 2020 (Principal Supervisor)
Research engineer and Postdoctoral Research Fellow
- Dr Shuang Liu, "Machine learning and remote sensing for environmental assesment", School of Mathematics and Statistics and UNSW Water Research Laboratory, since April 2023, (Joint Supervision with A/Prof Fiona Johnson)
- Danial Azam. ARC Basin Genesis Hub, University of Sydney, Cosupervision with Prof. Dietmar Muller (Jan 2018 - December 2020)
Research Interns
2024
- Vamshika Sutar, Indian Institute of Technology Guwahati, India (January - July 2024)
- Kartik Disawal, Indian Institute of Technology Goa, India (January - June 2024)
2023
- Mahek Vora, Indian Institute of Technology Guwahati, India (January - May 2023)
- Naman Jain, Indian Institute of Technology Delhi, India (May - July 2023)
- Akshat Shukla, Indian Institute of Technology Guwahati, India (January - April 2023)
2022
- Azal Khan, Indian Institute of Technology, Guwahati, India (January - April 2022)
- Saharsh Bharve, Manipal Institute of Technology, India (January - May 2022)
- Shirin Jain, Indian Institute of Technology, Guwahati, India (May - August 2022)
- Snigdha Jain, Indian Institute of Technology, Guwahati, India (May - August 2022)
- Janhavi Lande, Indian Institute of Technology, Guwahati, India (January - March 2022)
- Chaarvi Bansal, Birla Institute of Technology and Science, Pilani, Rajasthan, India (January - May 2022)
- Pranjal Singh, Indian Institute of Technology, Guwahati, India (January - April 2022)
- Gunjan Dhanuka, Indian Institute of Technology, Guwahati, India (January - April 2022)
- Suryansh Shrivastava, Indian Institute of Technology, Guwahati, India (January - April 2022)
- Pranshu Kandoi, Indian Institute of Technology, Guwahati, India (January - April 2022)
- Pandaya Pranshu, Indian Institute of Technology, Guwahati, India (January - April 2022)
2021
- Sweta Rathi, Indian Institute of Technology Guwahati, India (June - August 2021)
- Mukul Ranjan, Indian Institute of Technology Guwahati, India (June - August 2021)
- Amandeep Singh, Indian Institute of Technology Bombay, India (June - August 2021)
- Ritam Manabendra, Indian Institute of Technology Guwahati, India (June - August 2021)
- Anshul Negi, Indian Institute of Technology Roorkee, India (June - August 2021)
- Rishabh Sharma, Indian Institute of Technology Guwahati, India (June - August 2021)
- Sahil Bohra, Indian Institute of Technology Delhi, India (June - August 2021)
- Ayush Bhagat, Manipal Institute of Technology, India (April 2021 - June 2021)
- Venkatesh Kulkarni, Indian Institute of Technology Guwahati, India (November 2021 - February 2022)
- Sandeep Nagar, International Institute of Information Technology, Hyderabad, India (November 2021 - February 2022)
2020
- Ritij Saini, Indian Institute of Technology Bombay, India (December 2020 - February 2021)
- Aswin Krishna, Indian Institute of Technology Guwahati, India (December 2020 - February 2021)
- Prabhat Singh, Indian Institute of Technology Guwahati, India (December 2020 - February 2021)
- Jiaxin Yu, School of Mathematics and Statistics, UNSW Sydney, (December 2020 - February 2021)
- Jiaxi Zhao, School of Mathematics and Statistics, UNSW Sydney, (December 2020 - February 2021)
- Animesh Renanse, Indian Institute of Technology Guwahati, India (May 2020 - August 2020)
- Shaurya Goyal, Indian Institute of Technoritamlogy Delhi, India (May 2020 - August 2020)
- Yash Sharma, Indian Institute of Technology Roorkee, India (May 2020 - August 2020)
- Ashish Gupta, Indian Institute of Technology Delhi, India (May 2020 - August 2020)
- Manavendrasinh Maharana, Manipal Institute of Technology, India (Jan 2020 - June 2020)
- Animesh Tiwari, Indian Institute of Technology Guwahati, India (May 2020 - August 2020)
- Eshwar Nukala, Indian Institute of Technology Guwahati, India (May 2020 - August 2020)
- Arya Arya Indian Institute of Technology Jammu, India ( August 2020 - December 2020)
- Mahir Jain, Manipal Institute of Technology, India ( August 2020 - December 2020)
- Ayush Bhagat, Manipal Institute of Technology, India ( August 2020 - December 2020)
- Ayush Jain, Indian Institute of Technology Guwahati, India (May 2020 - August 2020)
- Divyanshu Singh, Indian Institute of Technology Guwahati, India ( August 2020 - December 2020)
- Kousik Rajesh, Indian Institute of Technology Guwahati, India (May 2020 - August 2020)
2019
- Aakarsh Yadav, Indian Institute of Technology, India (June 2019 - August 2019)
- Ashray Aman, Indian Institute of Technology Delhi, India (June 2019 - August 2019)
- Rishab Gupta, Indian Institute of Technology, India (June 2019 - August 2019)
2018
- Konark Jain, Indian Institute of Technology, India (May 2018 - July 2018)
- Arpit Kapoor, SRM Institute of Technology, India (June 2018 - August 2018)
- Ratneel Deo, University of the South Pacific, Fiji (December 2017 - February 2018)
- Wil Grebner, University of Sydney, Australia (February 2018 - June 2018)
My Teaching
Master of Data Science:
- ZZSC5836 - Data Mining and Machine Learning (Online), https://studyonline.unsw.edu.au/online-programs/master-data-science
- MATH5836 - Data Mining, Trimester 3: https://www.maths.unsw.edu.au/courses/math5836-data-mining Github repo: https://github.com/rohitash-chandra/dataminingMATH5836
Programming Bootcamp:
- Resources - code and exercises: https://github.com/rohitash-chandra/python-bootcamp
- Youtube Videos available