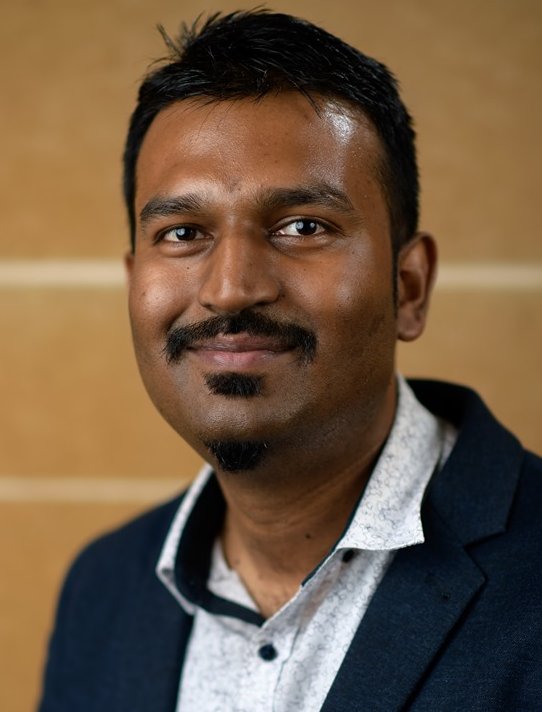
A recent paper by Dr Rohitash Chandra and colleagues has helped to close large gaps in the knowledge of environmental change over time, by harnessing the power of machine learning.
This cutting-edge research utilises machine learning to reconstruct precipitation for the last 250 million years based on coal deposits - contributing data that can guide scientists for expanded research to better understand the history of the planet.
To date, very little work has been undertaken to employ machine learning for deep-time geological data sets. Therefore, Dr Chandra and colleagues hope that this can develop into a multidisciplinary field, where artificial intelligence and machine learning methods can facilitate a more comprehensive understanding of the evolution of the planet.
Dr Chandra tells us about the research behind the paper.
----------
Who was involved in the research for your paper, Precipitation reconstruction from climate-sensitive lithologies using Bayesian machine learning?
I led the research paper which was conceptualised by Prof. Dietmar Muller who is an Earth Scientist. I worked with Prof. Sally Cripps in model development and Dr Nathaniel Butterworth for the visualisation of results. This is a multidisciplinary research effort between UNSW and the University of Sydney.
What is the central finding of the paper?
The geological and climate history of the planet is a complex puzzle to solve given sparse, limited and noisy datasets. The complexity increases when we go further back in time in millions of years ago covering supercontinents such as Pangea and plate tectonics that shaped the Earth we know today.
We used machine learning and statistical methods to provide inference by estimating the unknown data, which consist of climate-sensitive sedimentary deposits such as coal and evaporites like salt and gypsum, deposited over the last 250 million years. The presence of coal implies the presence of particular types of forests back in time. The distribution of these deposits is in turn linked to the distribution of rainfall back in time that ranges in millions of years.
Our model estimates the missing deposit data and uses it to estimate precipitation for 11 time-slices over the last 250 million years. Our model has been trained using precipitation data generated from global circulation models for two time periods in the Miocene and Eocene, 15 and 34 million years ago.
We know about the presence of coal and glacial deposits back in the time given fossil records. However, the records are sparse and not complete and different methods are needed to estimate if there is coal or glacial deposit in an area based on topographical features such as elevation, distance to the shoreline and prior knowledge such as the latitudinal range of climate belts.
What are the main implications of your findings?
Paleoclimatology entails the study of Earth's climate for millions of years that gives an understanding of the history of the planet along with diverse lifeforms. Our work has been the first of its kind to visualize the surface environmental conditions on a series of maps which can be used by Earth scientists to have a better understanding of environmental change through geological time.
What does your research add to what is already known on this topic?
The disintegration of supercontinent Pangea is well known and there are sophisticated simulations about it that use the knowledge from the fossil records and other geological features. We have a rough idea of the evolution of surface topography since then.
However, we do only have limited information about environmental features, such as forests or deserts due to missing data. Our method has reconstructed, along with model development, missing data based on prior knowledge and sparse information using machine learning. Moreover, we have estimated the precipitation with a series of maps that covers the disintegration of Pangea to the evolution of the major continents we know today.
Scatter plots for establishing the marginal relationship of the spatial covariates with respect to the precipitation in Miocene (blue) and Eocene (red) combined.
Is there anything you find particularly surprising about your research?
Our research had the goal to further validate and extend the knowledge of the experts using modelling and simulation powered by machine learning. Our maps connect the missing pieces of information back in time. We also need to note that our maps cover the Jurassic era and there have been drastic changes in the evolution of biological life forms across the time slices shown in our maps. This opens the road for further research in a multidisciplinary field that connects ancient climates to lifeforms.
What do you identify as the main impact of your research?
This is ground-breaking research that contributes data (in terms of paleo maps) that can guide scientists for further research in better understanding the history of the planet. So far, there has been very little work done in connecting machine learning to deep-time geological data sets. We hope that this develops into a multidisciplinary field where artificial intelligence and machine learning methods enable Earth and climate scientists to get a better understanding of the evolution of planet Earth.
Snapshot of the simulation:
Below: Maps of predicted probability of coal deposits. The presence (observation) of a deposit used as training data is given by the orange points on the grid