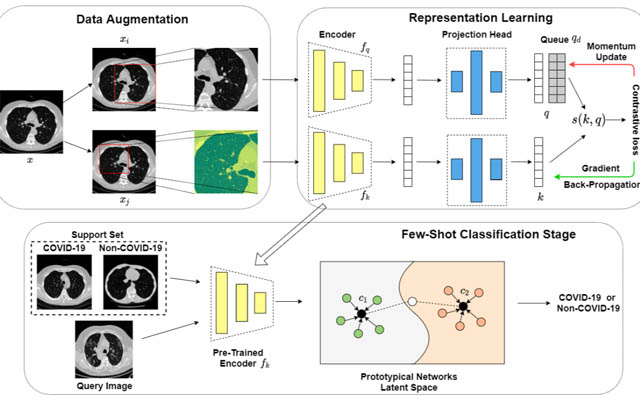
Associate Professor Lina Yao and her research team propose a new deep learning algorithm for the automated diagnosis of COVID-19, which only requires a few samples for training. The researchers first use contrastive learning to train an encoder, and then validate the efficacy of the proposed model in comparison with other competing methods. The results demonstrate the superior performance of their model for the accurate diagnosis of COVID-19 on chest CT images. The study appears in the prestigious journal Pattern Recognition.
This work, collaborating with Stanford University, Lehigh University, United Arab Emirates University, provides a plausible solution to enable and improve the earlier diagnosis accuracy of COVID-19 amid the pandemic given the very limited available CT images.
Grad-CAM (Selvaraju et al., 2017) visualizations for learned features from several baseline methods. Top line of above CT images is the original image set, followed by our methods, our methods with ImageNet pre-train, DenseNet-121 and ResNet-50. It is obviously that our method can learn lung's features better.
Journal Reference:
“Momentum Contrastive Learning for Few-Shot COVID-19 Diagnosis from Chest CT Images” (SJR Q1, CORE A*, Impact Factor: 7.196)
Picture source: www.researchgate.net.