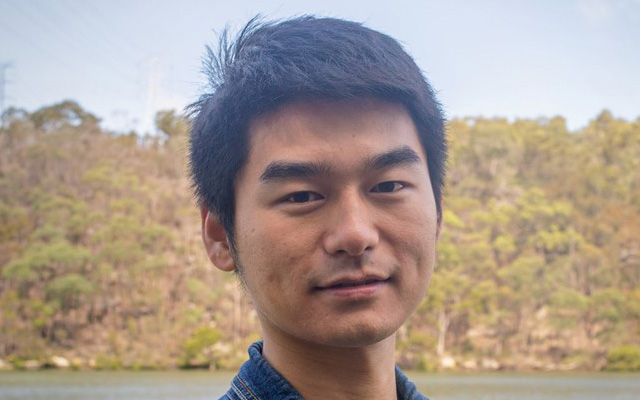
Bio: Mr. Lei Bai is a PhD student supervised by Scientia A/Prof. Lina Yao and Prof. Salil Kanhere with School of Computer Science and Engineering. His research interest lies in Machine Learning and Data Mining, with focus on spatio-temporal learning in the multi-modal correlated time series. He has published 13 peer-reviewed research papers on flagship AI conferences and prestigious journals (e.g., NeurIPS, IJCAI, UbiComp, and KDD). He was awarded 2020 Google PhD Fellowship in Machine Learning. The Google PhD Fellowship Program was created to recognize outstanding graduate students doing exceptional and innovative research in areas relevant to computer science and related fields.
Mr Lei Bai’s research focusses on developing discriminative, robust, and end-to-end machine learning solutions to represent the multi-modal correlated time series and conduct corresponding predictive/classification tasks. This is challenging because data from different entities keep involving with time and interacting with each other and the ever-changing environment. Traditional data mining techniques are overwhelmed due to the huge number, volume, and complex dependencies of the data, which make it urgent to develop new algorithms for processing these large-scale data streams.
He is tackling three major challenges: (1) Complex spatial and temporal correlations: real-world application/systems normally contain massive entities (e.g., sensors) that interact with each other, resulting in multiple correlated data streams. The data exhibits inter-dependencies among different entities (spatial correlations) and intra-dependencies between different time periods, which are complex in three ways. First, the affinity among different streams is unclear. Second, temporal correlations are diverse, including long-term, short-term, and periodic temporal patterns. Third, the spatio-temporal correlations are dynamic due to the evolving priority of time series. Developing a unified and discriminative model to capture these correlations comprehensively and accurately is the foremost challenge.
(2) Multi-modal heterogeneous data: another problem lays in the area is the multi-modal and heterogeneity. Real-world systems normally interact with and are influenced by other systems continually. Thus, various extraneous factors may be correlated with the target systems, such as the time-meta, weather, and traffic. These data may have totally different types, scales, and dimensions. Effectively distilling and fusing the essential knowledge while filtering out the irrelevant information from these correlated multi-modal time series is challenging.
3) Pattern drift and divergence: traditional machine learning algorithms assume the data follows independent and identically distribution, which does not hold in the real-world correlated time series. The patterns in the data may change with time and show disparities in different sources. The drift and divergence would change the spatial and temporal patterns dramatically and make existing algorithms invalid. Thus, developing a robust model that can adapt to the pattern shifts is an important challenge.
Lei’s research has the huge potential to boost and revolutionize a wide array of real-world applications. With the fast evolution of mobile internet and remote sensing technologies, abundant real-time data from different devices are generated and collected with time and source tags. Mining the knowledge from these multi-modal correlated time series data is valuable and essential for understanding the system and making intelligent decisions in various applications, such as human behaviour analysis, epidemic transmission control, smart transportation, and neuroscience.