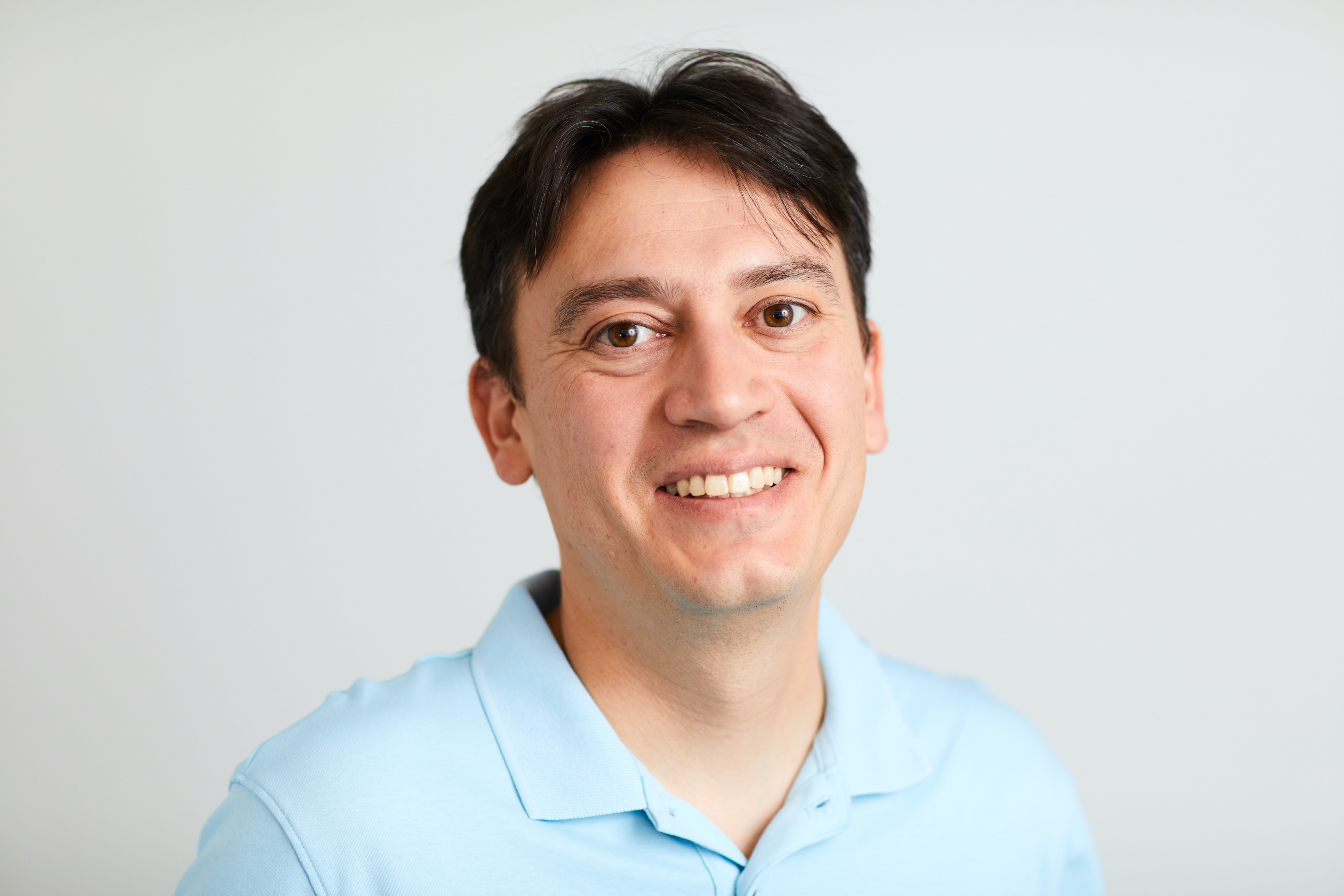
Associate Professor Gustavo Batista
- 2016. Habilitation. Computer Science. University of São Paulo at São Carlos, Brazil.
- 2003. Doctor of Philosophy (PhD). Computer Science. University of São Paulo at São Carlos, Brazil.
- 1997. Master in Science (MSc). Computer Science University of São Paulo at São Carlos, Brazil.
- 1994. Bachelor (BS). Computer Science. São Paulo State University, Brazil.
I joined UNSW as an associate professor in 2018, after working for more than ten years for the University of Sao Paulo (USP). During 2010-2012, I was a visiting researcher at the University of California, Riverside (UCR) working in the prof. Eamonn Keogh's laboratory.
During my stay at UCR, I continued my work with time series analysis, particularly developing methods for classification and clustering of time-oriented data. In conjunction with Dr Keogh, I proposed the first time series distance invariant to complexity and speed-up techniques to compare massive amounts of time series data under warping.
More recently, I have worked with data streams, particularly with classification with label latency and proposed efficient unsupervised methods to detect concept drifts as well as to learn in the presence of these changes in the data distribution.
My research is motivated by applying Machine Learning in practice. My approach is to work on challenging applications that help my students and me to identify gaps in the literature or assumptions in the state-of-the-art that do not hold for our applications. This research approach often leads to contributions both in Computer Science as well as the application areas.
One instance of such an approach is the challenge of incorporating classification algorithms on embedded devices. For example, I have developed lightweight models that can run in environments with severe power restrictions such as satellites and sensors. One notorious application is the development of sensors to classify insects in flight automatically, allowing the creation of surveillance systems for disease vectors, invasive species and pests. I have also developed EmbML, a Machine Learning tool to convert sickit-learn and Weka classifiers into C++ code crafted to run into low-power microcontrollers, such as ones found in the Arduino family.
In the last years, I have actively worked in the area of Machine Learning Quantification, developing new algorithms to count events accurately. These recent developments have led to the proposal of a novel Data Mining task known as One-class Quantification as well as a family of efficient quantification algorithms.
The impact of my research can be measured by the number of recent papers citing my research articles. According to Google Scholar, my paper have more
than 9,000 citations, with more than 1,000 citations in 2020.
- Publications
- Media
- Grants
- Awards
- Research Activities
- Engagement
- Teaching and Supervision
Grant funding as principal investigator
- 2017 – 2019: FAPESP e-Science Research Grant. Intelligent Traps and Sensors: an Innovative Approach to Control Insect Pests and Disease Vectors. $55,000.
- 2016 – 2019: USAID Combating Zika and Future Threats Grand Challenge. An Intelligent Trap and Mobile Application to Motivate Local Mosquito Control Activities. $500,000.
- 2017 – 2019: CNPq Research Fellow. Novel Approaches in Machine Learning Applied to Automatic Insect Recognition. $25,000.
- 2015 – 2016: Google LA Research Award. Controlling Dengue Fever Mosquitoes using Intelligent Sensors and Traps. $24,000.
- 2012 – 2014: FAPESP Research Grant. Complexity-invariance for Classification, Clustering and Motif Discovery in Time Series. $30,000.
- 2013 – 2015: FAPESP-CALDO International Cooperation Grant. Research on Geospatial Marine Biology Data Mining using Time Series, Text Mining and Visualization (with Stan Matwin co-PI for NSERC). $20,000.
- 2013 – 2015: FAPESP-CNPq Research Grant. Intelligent Sensors for Controlling Agricultural Pests and Disease-vector Insects. $55,000.
- 2014 – 2017: CNPq Universal Research Grant. Real-time Monitoring of Insect Pests in Agriculture and the Environment. $25,000.
- 2014 – 2017: FAPESP New Frontiers Grant. Time Series Classification Algorithms Applied to Embedded Systems. $30,000.
- 2007 – 2009: FAPESP Research Grant. Machine Learning and Class Imbalance. $10,000.
- 2020. Best Research Paper Award. IEEE International Conference on Data Science and Advanced Analytics (IEEE-DSAA).
- 2017 – 2020. Research Fellow, level 2. National Council for Scientific and Technological Development, CNPq.
- 2014 – 2017. Research Fellow, level 2. National Council for Scientific and Technological Development, CNPq.
- 2015 – 2016. Google Research Award in Latin America. Google Inc.
- 2012. Best Research Paper Award. ACM SIGKDD Conference on Knowledge Discovery and Data Mining (ACM-KDD).
I have worked in Machine Learning during my entire career. My main contributions to the field are the following:
Quantification: I have developed counting algorithms that are robust to changes in data distributions that occur in real-world applications. The algorithms developed by my research group, such as the ones of the DyS family are among the most accurate ones. We recently developed an ultra-fast counting algorithm which performs similarly to the state-of-the-art. This algorithm received the Best Research Paper Award at DSAA-2020.
Time Series Mining: I have created algorithms to classify and cluster time-oriented data under different invariances such as warping. Such developments lead to the UCR suite, a framework for time series matching under warping that received the KDD Best Research Award in 2012. More recently, we further improved the search speed of the UCR suite, creating the UCR-USP suite. I also proposed the first time series distance invariant to complexity.
Class imbalance: My initial research involved the development and assessment of methods to deal with imbalanced class data. My research focused on discussing the challenges of learning with imbalanced data, including the scenarios in which skewed distributions would impose difficulties for classifiers. My articles figure among the most cited in the topic, including the ACM SIGKDD paper of 2004 with more than 2,500 citations.
Missing data imputation: During my PhD, I worked with data preprocessing techniques, including missing data imputation methods. I developed and demonstrated the use of k-nearest neighbour (k-NN) as a flexible technique for missing data imputation and demonstrated its efficacy comparing to other techniques in the state-of-the-art. k-NN is currently one of the most used imputation algorithms due to its simple implementation, ability to deal with missing data in multiple attributes and capacity to work with continuous and discrete features.
My Research Supervision
- Tiago Pinho da Silva, PhD student: Election Forensics: Detecting Irregularities in Electoral DataUnder Spatial Non-Stationarity.
- Antonio Parmezan, PhD student: Hierarchical Classification of Data Streams.