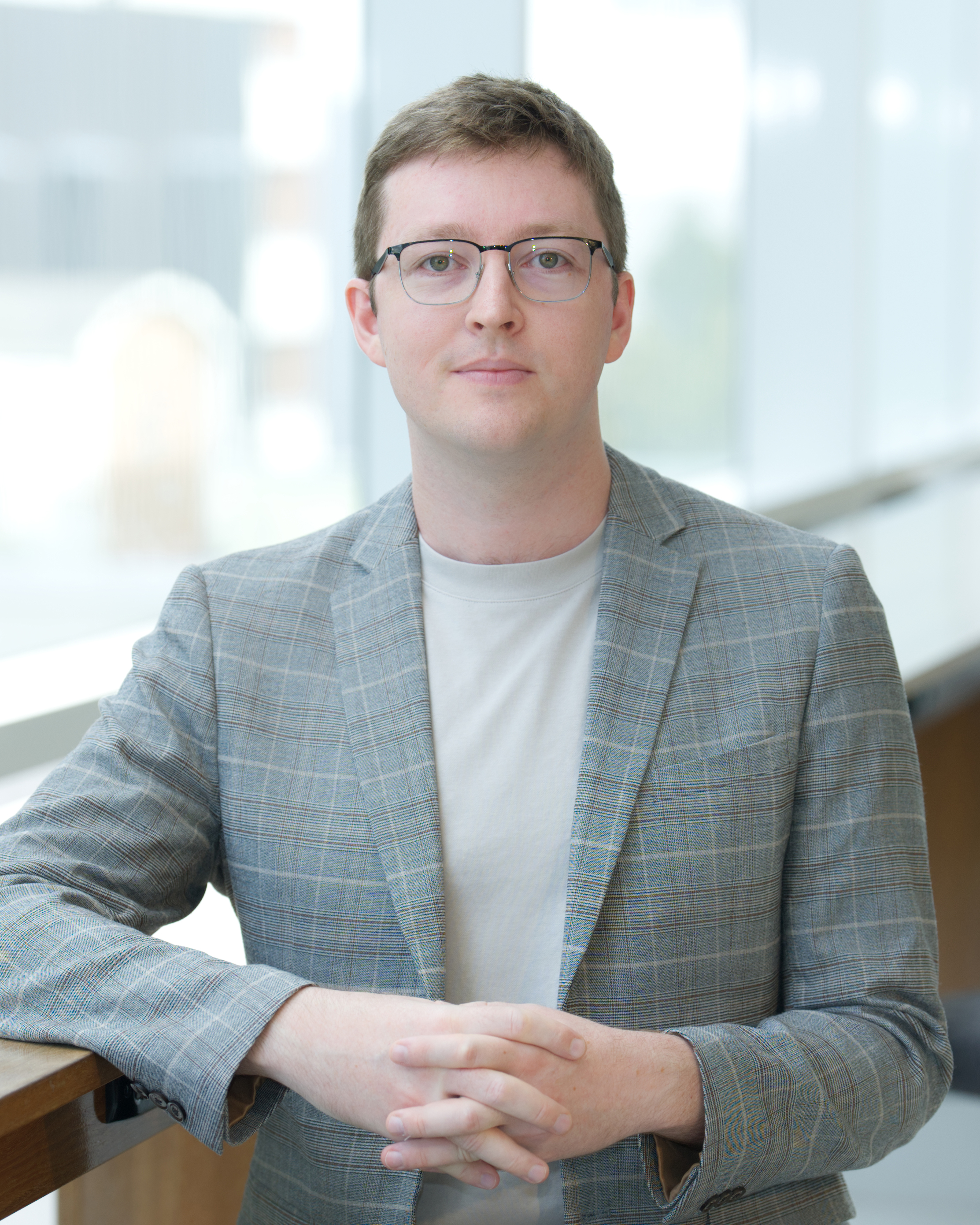
Dr Patrick Laub
Patrick Laub is a lecturer at the UNSW School of Risk and Actuarial Studies. His teaching covers artificial intelligence and machine learning courses, with a focus on risk and insurance applications. He holds a PhD in computational applied probability and degrees in software engineering and mathematics. Patrick's research focuses on computationally challenging problems in actuarial data science.
- Publications
- Grants
- Awards
- Research Activities
- Engagement
- Teaching and Supervision
- Media
Patrick's recent research topics include the Hawkes Processes, Approximate Bayesian Computation, and Empirical Dynamic Modelling. Patrick's joint PhD in computational applied probability was completed between the University of Queensland and Aarhus University. For further information, see https://www.patrick.laub.au.
My Teaching
Since 2022, Patrick developed and taught new courses on artificial intelligence & deep learning and their applications to risk and insurance (ACTL3143 and ACTL5111). He also teaches the course Statistical Machine Learning for Risk and Actuarial Applications (ACTL5110).