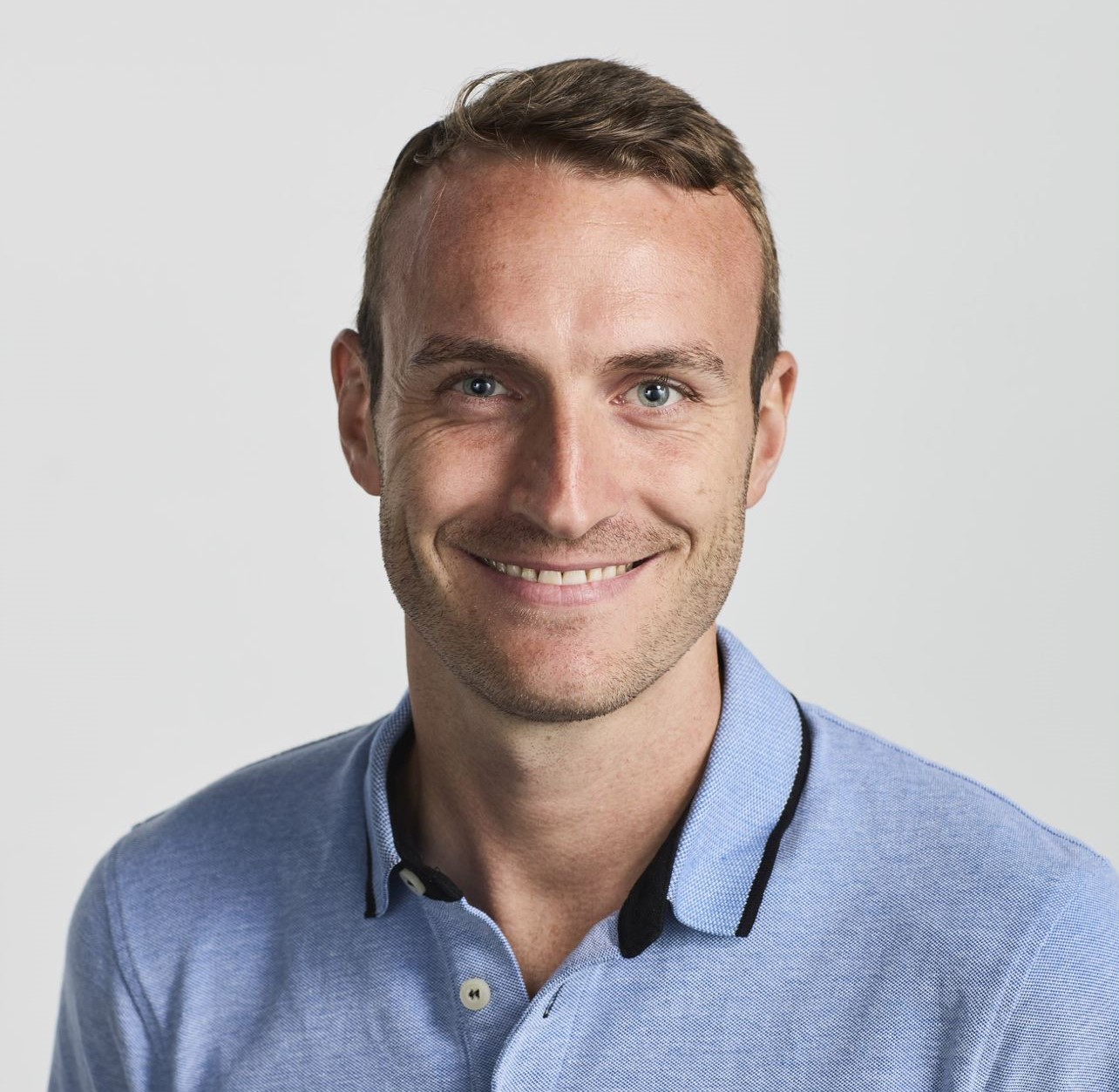
Dr Tom Stindl
Tom Stindl is a statistician and Lecturer in the School of Mathematics and Statistics. He is interested in statistics and computing. His recent research topics include Hawkes Processes, Statistical Inference, and Computational Statistics. Tom's Ph.D. was on statistical inference for self-exciting point processes under the supervision of Dr. Feng Chen at UNSW Sydney.
- Publications
- Media
- Grants
- Awards
- Research Activities
- Engagement
- Teaching and Supervision
- Feng Chen, Tom Stindl, William Dunsmuir, Jiancang Zhuang. "Inference for Hawkes processes with challenging data", Australian Research Council, Discovery Project. 2024 - 2026, $463,452.
Early Career Teaching Excellence - Faculty of Science
Research Aims
My core research is in point process models and their application in a range of disciplines. Currently, my research is focused on the following themes:
- Statistical inference for self-exciting point processes
- Application of self-exciting point processes to finance, seismology, crime, and bushfires
- Financial data modeling
Research in Detail
My research focuses on methods to perform efficient statistical inferences for point process models, with a particular focus on the renewal Hawkes process and its marked and multivariate variants.
Potential Projects:
Limit Order Book Modeling: Develop a point process model for order flows in limit order books. More specifically, a Hawkes process with a state-dependent factor with potential state observations including observed imbalance or spread among other economic variables. The project would develop computationally efficient methods for estimation using direct MLE or EM algorithms including methods for goodness-of-fit assessment. These methods would then serve to conduct an empirical study on different ASX stocks.
Bayesian estimation for ETAS model with application to seismology: The ETAS model has been successfully modeled using likelihood-based algorithms such as MLE and EM algorithms. However, forecasts based on frequentist approaches fail to account for the uncertainty in the estimates. By employing a Bayesian approach the parameter uncertainties can be explicitly accounted for in the forecast. This project will continue the development of the Bayesian framework for estimating the ETAS model and developing the appropriate software to implement the methods. These methods would be used to fit an earthquake catalog and perform forecasts of future seismicity.
Non-parametric estimation for ETAS model with application to crime: Crime events such as burglaries and gang violence cluster in both time and space due to the crime-specific patterns of criminal behavior. A self-exciting space-time point process is well suited to model this clustering behavior. In this project, we will apply a non-parametric space-time point process with a temporal background process that renews on each background event.
My Research Supervision
PhD Student(s):
- Jason Lambe (2024 - ; joint with Feng Chen)
MRes Student(s):
- Zhe Han (2020 - 2022 ; joint with Feng Chen): Modulated renewal Hawkes process
Honours Students:
- Miah Panovrakos (2024)
- Oden Petersen (2024)
- Claire Xue (2023 - ; joint with David Khoury)
- Thomas Lee (2021, joint with Feng Chen): Ergodicity of renewal Hawkes processes
Masters Students:
-
Xiaowen Liang (2020): Modeling intraday extreme negative financial returns using renewal Hawkes processes
-
Zhipeng Dai (2021): Approximate Bayesian computation for self-exciting point processes
-
Yuxin Song (2021): Modeling Left- and Right-tailed extreme return using point processes
-
Ke Zhu (2021): Point processes with gamma distributed self-excitation
-
Sarah Akari (2022): Tail risk estimation using an extreme value approach with covariates
-
Zhuoyu Zhou (2022): Using Pearson IV and Generalized Hyperbolic distributions to estimate tail risk related measures
-
Dongyue Lu (2022 - ): Self-exciting point processes with inhibition
-
Daniel Ng (2022 - ): Machine learning approach to predicting the mid-point/fair value of BTC-perpetual swap
My Teaching
Courses recently taught:
- MATH3821 - Statistical Modelling and Computing (T2 2019, T2 2020)
- ZZSC5905 - Statistical Inference for Data Scientists (H6 2019)
- MATH5905 - Statistical Inference (T1 2020)
- MATH2099 - Mathematics 2B (Statistics component, T2 2020)
- MATH2859 - Probability, Statistics, and Information (T2, 2020)
- DATA3001 - Data Science and Decisions in Practice (T3, 2020)
- MATH3811/MATH3911 - Statistical Inference (T1, 2022)
- MATH3841 - Statistical Analysis of Dependent Data (T2, 2023)