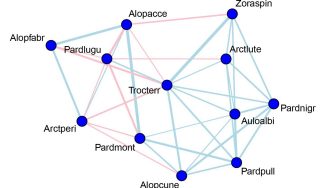
Multivariate abundance data are abundances collected simultaneously for many taxa (species, orders, functional groups...). This type of data is commonly collected in ecology and the environmental sciences, and has been collected and analysed in thousands of publications. There are many possibilities for significant contributions to this field, using a more rigorous model-based approach to analysis.
- Projects
- Software
- Penalised likelihood techniques for model-based hierarchical classification (Gordana Popovic).
- Design based inference for mixed models of multivariate data (Loic Thibaut).
- Developing the mvabund package for model-based analysis of multivariate abundance data (Loic Thibaut, Alice Wang).
- Fast methods for fitting latent variable models to multivariate data (with Francis Hui, Sara Taskinen, and John Ormerod).
- Model-based methods for vegetation classification (Mitchell Lyons with David Keith, Jane Elith, Stuart Phinn).
- Modelling species interaction (Gordana Popovic).
- The PIT-trap for residual resampling of non-normal data (with Alice Wang).
- Model-based approaches to multivariate analysis, in the mvabund package (Alice Wang, Ulrike Naumann, Stephen Wright)
- These methods resolve some undesirable power properties seen in algorithmic approaches to analysis (Alice Wang, Stephen Wright)
- Incorporating species traits in analyses to explain interspecific variation in environmental response, the "fourth corner problem" (Alex Brown, with Bill Shipley and Trevor Hastie)
- Finite mixture modelling to cluster species based on their environmental response (Francis Hui, with Scott Foster and Piers Dunstan).
Software for multivariate analysis in ecology, via visualizing and modelling key data properties (in particular, the mean-variance relationship). This avoids undesirable properties of algorithmic approaches (Warton et al 2012). The package has (model-based) methods for hypothesis testing, including traits in models to explain interspecific variation, and predictive modelling tools via the LASSO.
Project ideas
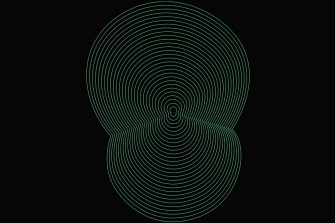
New model-based approaches for analysis of high-dimensional abundances
Many datasets are high dimensional, i.e. they contain many variables compared to the number of independent observations. Multivariate abundance data in ecology is our primary focus, although data with some similar properties arise elsewhere (bioinformatics, portfolio theory). A key challenge is developing methods that can make valid multivariate inferences when there is little information that can be used to characterise correlation between variables. Particular problems currently of interest: how to model dispersion in multivariate data; correcting for false discovery rate in resampling algorithms for non-normal data; specifying a realistic marginal model for % cover data; model-based hierarchical classification for non-normal data.
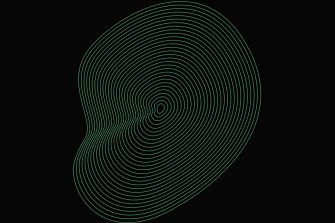
Community-level modeling - (multivariate) spatial models in ecology
It is of key interest to understand how ecological communities respond to their environment, in order to understand their potential response to changes in their environment (due to climate change, urbanization, or other environmental impacts). One important issue that has not been considered in detail is that of response and predictor variables available at different spatial resolutions. A second set of issues revolves around the analysis of opportunistic “presence-only” records, often the best available data, especially for rare species. In this project you will develop and evaluate methods of species distribution modelling, initially focussing on the climate response of shrub and tree species in the Sydney basin. Key methodological challenges will involve: addressing the issue of variables available at different spatial resolutions; generalising single-species presence-only models so that they can be used to simultaneously model a whole community of species in an efficient way.