Study with us
Looking for project ideas? See below.

Are you interested in joining Eco-Stats? Below are some project ideas - ranging from methodological to applied, depending on your interests and expertise, or of course be a mixture of both. Methodological projects will focus on developing and evaluating new methods of data analysis, and exploring their properties. Applied projects typically involve collaborating with ecologists working at UNSW and elsewhere, using modern statistical methods to address important research questions arising in ecology.
Not 100% sure the ecology focus is for you? Firstly, note that prior experience in ecology (while useful) is not required, just a willingness to learn! But secondly, problems we deal with are encountered in other disciplines also (e.g. bioinformatics, epidemiology) so skills you hone in our group enable all sorts of interesting research careers. Eco-Stats Alumni have gone on to work as biostatisticians at top universities in London, academics in Statistics and Computer Science departments, and research positions at medical institutes.
Eco-Stats researchers are based in the School of Mathematics and Statistics at UNSW, but are also affiliated with the Evolution & Ecology Research Centre and the Centre for Ecosystem Science. As such, it is possible to do an Eco-Stats project while being enrolled through any of these organisational unit
Project ideas

Efficient algorithms for fitting latent variable models
Models including latent variables are invaluable in ecology for a number of applications - not just for models with surveys with multiple sampling units, but recently we have been interested in their application for visualising and modelling multivariate data, and for accounting for errors in predictor variables. How can we fit such models more efficiently? We will explore a number of options centered around extensions of the Monte Carlo EM algorithm and efficient Laplace and variation approximation algorithms.
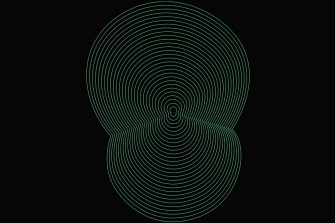
New model-based approaches for analysis of high-dimensional abundances
Many datasets are high dimensional, i.e. they contain many variables compared to the number of independent observations. Multivariate abundance data in ecology is our primary focus, although data with some similar properties arise elsewhere (bioinformatics, portfolio theory). A key challenge is developing methods that can make valid multivariate inferences when there is little information that can be used to characterise correlation between variables. Particular problems currently of interest: how to model dispersion in multivariate data; correcting for false discovery rate in resampling algorithms for non-normal data; specifying a realistic marginal model for % cover data; model-based hierarchical classification for non-normal data.
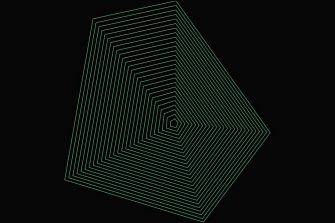
Advances for errors-in variables modelling
Often predictor variables (in ecology and elsewhere) are measured with error, and failing to take this into account biases estimates of the fitted model, and often, subsequent predictions. We have been developing easy-to-use algorithms for modelling such data, having initially focussed on generalised linear models for data with measurement error that is independent across observations. Important extensions include: how to extend to spatially correlated measurement error? How to generalise to handle general predictive models (beyond GLM)?
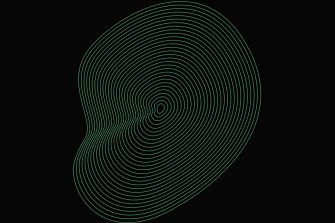
Community-level modeling - (multivariate) spatial models in ecology
It is of key interest to understand how ecological communities respond to their environment, in order to understand their potential response to changes in their environment (due to climate change, urbanization, or other environmental impacts). One important issue that has not been considered in detail is that of response and predictor variables available at different spatial resolutions. A second set of issues revolves around the analysis of opportunistic “presence-only” records, often the best available data, especially for rare species. In this project you will develop and evaluate methods of species distribution modelling, initially focussing on the climate response of shrub and tree species in the Sydney basin. Key methodological challenges will involve: addressing the issue of variables available at different spatial resolutions; generalising single-species presence-only models so that they can be used to simultaneously model a whole community of species in an efficient way.
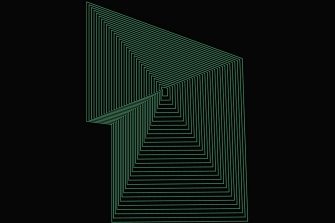
Allometry - extending tools for inference about size
Allometry is the study of how one size variable scales against the other. Typically methods related to principal components are used for analysis, although in low dimensions (usually 2-3D), and with the primary focus being inference about model coefficients rather than data reduction. Two problems yet to be adequately addressed in the literature: how to make inferences about allometric lines whose coefficients are a function of continuous covariates; how to generalise inferential methods to relax the independence assumption across points to handle phylogenetic relationships between variables.