Bayesian and Monte Carlo methods
Advancing science through computational statistics.
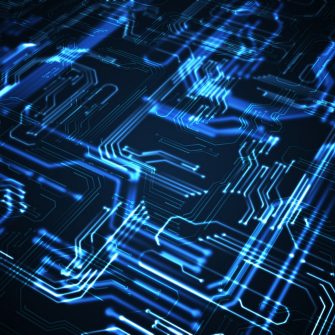
“The fundamental problem of scientific progress, and a fundamental one of everyday life, is that of learning from experience. Knowledge obtained in this way is partly merely description of what we have already observed, but partly consists of making inference from past experience to predict future experience."
Sir Harold Jeffreys, Theory of Probability
The Bayesian approach to statistical modelling uses probability as a means to quantify the beliefs of the observer about the model parameters, given the data observed. Computationally intensive methods such as Markov chain Monte Carlo have facilitated the application of Bayesian methods to a diverse range of fields, including archaeology, ecology, engineering, medicine, epidemiology and biostatistics. Trans-dimensional Markov chains permit the Markov chain to traverse through varying dimensions over time. The simultaneous exploration of model and parameter space is particularly useful in the context of Bayesian model determination and model averaging. Performance of Markov chains has long been a contentious issue, and recent progress in adaptive algorithms and exact or perfect sampling have provided exciting avenues of research.
Specific research interests of this group include the application of Bayesian methods, simulation via trans-dimensional Markov chains, stochastic simulation in the absence of likelihoods (approximate Bayesian computation), Monte Carlo methods for rare event simulation, and statistical analysis of financial time series data using heavy-tailed distributions.
Group members
About the Bayesian Statistics and Monte Carlo Methods group
The group has strong ties with the School of Economics through Robert Kohn, and with the Australian Graduate School of Management through Sally Wood. There are a number of PhD and postdoctoral researchers between the schools.
Research in the group is sponsored by the Australian Research Council.
Some ongoing projects
Approximate Bayesian computation
There are many applications in which the likelihood for a model is not known, or is computationally prohibitive to evaluate, but where it is possible to efficiently simulate data. The group is currently active in developing so-called approximate Bayesian computation algorithms capable of simulating from the posterior distribution implied by such models.
Automating trans-dimensional Markov chains
Trans-dimensional samplers, such as the reversible jump algorithm, require specification of both within- and between- model transitions. Efficient transitions are difficult to find for generic models, in particular for the between-model moves. The group is currently active in developing techniques and algorithms to automate the selection of the most efficient transitions for such samplers.
Bayesian inference and computation for complex regression models
The Bayesian statistics and Monte Carlo methods group is also active in researching Bayesian approaches to inference and computation for complex regression models. Some particular interests of group members are flexible simultaneous modelling of mean and variance functions, Bayesian hierarchical modelling of data from gene expression studies and Bayesian hierarchical modelling of data sets arising in meteorology and environmental science.
MCMC and sampling methodology
The group is interested in the development of novel methodology concerning sampling algorithms. This includes the assessment of convergence properties and diagnostics, perfect or exact simulation, sequential Monte Carlo methods, model selection and transdimensional sampling schemes.
Rare event probability estimation
Rare event simulation and modelling is critical to our understanding of high-cost hard-to-predict events such as nuclear accidents, natural disasters, and financial crises. Quantitative analysis of such high impact events demands the accurate estimation of the probability of occurrence of such rare events. In realist models, this probability is very hard to estimate, because exact simple analytical formulas are not available. Our goal is to develop effective Monte Carlo methods that will be able to reliably and accurately estimate rare-event probabilities in a variety of contexts.
Financial time series modelling
Bayesian computational methods are also extensively used for statistical analyses of financial time series data and insurance data, using heavy-tailed distributions via scale mixture density representations. In particular, focus has been on non-linear heteroskedastic stochastic volatility models in financial applications.