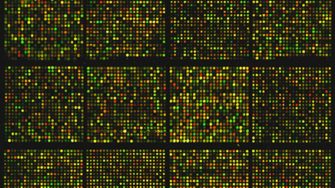
If you are an Advanced Mathematics or Advanced Science student, then Honours is built into your program. For all other students, if you are keen on mathematics and statistics and have achieved good results in years 1 to 3, you should consider embarking on an Honours year.
For information about doing Honours in Statistics, see the Honours Page.
Honours Coordinator - Statistics
If you have any questions about the Honours year, please don't hesitate to contact our Statistics Honours Coordinator Gery Geenens via the details below.
Statistics project areas
The following are suggestions for possible supervisors and Honours projects in Statistics. Other projects are possible, and you should contact any potential supervisors to discuss your options.
-
- Analysis of extremes: theory, computations, environmental applications
- Big and Complex Data analysis
- Kernel Density Estimation
- Multilevel and Markov Chain Monte Carlo Methods for Rare-Event Simulation
- Monte Carlo Methods in Network Reliability
- Mathematical finance
- Bayesian deep learning with variational inference
- MCMC sampling
- Bayesian data imputation for medical and climate data
- Inference and applications of stochastic processes, especially point processes
- Bayesian statistics
- Markov chain Monte Carlo
- Statistical dependence: modelling, quantification and analysis
- Copula modelling: theory and applications
- Nonparametric and semiparametric estimators for probability densities and regression functions
- Sports analytics (mainly football/soccer)
- Stochastic differential equations
- Social network analysis
- Statistical computing
- Surveys and sampling
- Dependence Measures
- NeuroImaging
- Big Data and Internet of Things
- Statistical Inference for Complex Random Vectors
- General theory of stochastic processes and its applications in financial mathematics
- Fast and efficient model selection for high-dimensional data
- Development of efficient estimation and sampling algorithms for random graphs and spatial point processes
- Development of model compression methods for deep neural networks.
- Machine learning prediction of bluebottles presence along the Australian coast
- Statistical and Experimental approach to estimation of tyres in a stockpile
- Deep learning models for spatio-temporal data
- Statistical methods for the analysis of count data with applications to epidemiology and population health data
- Generative modelling and sampling methods (incl. sampling for Bayesian inference, sequential Monte Carlo)
- Stochastic differential equations and their applications
- Analysis of modern machine learning techniques
- Stochastic hydrology
- Wavelet methods in non-parametric inference
- Latent Variable Models
- Financial modelling
- Fractional Brownian motion
- Bayesian computational techniques
- Models of extremes of climate processes
- Genetic epidemiology
- Analysis of capture-recapture data and estimation of animal abundance
- Measurement error modelling
- Non-parametric smoothing
- Ecological statistics: see UNSW Eco-Stats Opportunities
- High-dimensional data analysis
- Species distribution modelling
- Time series analysis
- Functional data analysis
- Analysis of extremes: theory, computations, environmental applications