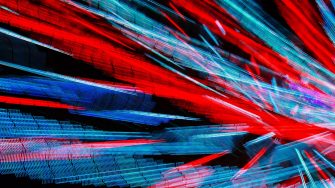
The Honours in Quantitative Data Science is intended for students who have completed the Quantitative Data Science stream in program 3959, the Bachelor of Data Science and Decisions. It is also open to other students from other programs with a suitable mix of undergraduate courses.
Below you can find some specific information about Quantitative Data Science Honours.
For more general info about doing Honours in Quantitative Data Science, see the Honours Page.
Honours Coordinator - Quantitative Data Science
If you have any questions about the Honours year, please don't hesitate to contact the Honours Coordinator.
Honours Coordinator- Quantitative Data Science
Dr Rohitash Chandra
B: rohitash.chandra@unsw.edu.au
T: 0413071839 or 02 9385 7111
Office: H13 Lawrence East 2053
Quantitative Data Science project areas
The following are suggestions for possible supervisors and honours projects in Quantitative Data Science. Other projects are possible, and you should contact any potential supervisors to discuss your options.
-
- Extreme value theory
- Bayesian neural networks
- Deep learning
- Machine learning
- Earth and climate data science
- Spatio-temporal data analysis
- Inference with incomplete data
- Statistical computation
- Approximation properties of neural networks
- Nonparametric and semiparametric density estimation
- Nonparametric and semiparametric regression, in particular binary regression
- Functional data analysis
- In collaboration with Spiral Blue, Detecting maritime vessel anomalies using k-means clustering
- Optimization based decision-making under data uncertainty
- Data-driven robust optimization
- Global optimization and data classification
- Social network analysis
- Analysis of blockchain data
- Statistical computing
- Dependence measures
- Neuro-imaging genetics
- Data science for IoT
- Optimisation methods in machine learning
- Optimisation under data uncertainty
Raymond Louie (Co-supervisor; UNSW Computer Science & Engineering)
- Statistical analysis of molecular data and applications in vaccine and drug design
- Statistical modelling of biological systems
- Machine learning
- Making sense of naturalistic driving study data
- Inference about expectiles
- Optimal capital allocation
- Projection methods for machine learning
- (Higher-order) Voronoi diagrams for data analysis
- Conic programming and semidefinite optimisation for data science
Moninya Roughan (co-supervisor)
- Oceanography
- Big data and time series
- Correlated variables
Amandine Schaeffer (co-supervisor)
- Environmental drivers of marine heatwaves
- Understanding biological productivity in the ocean: statistical model from in-situ glider observations
- Bayesian inference
- Computational statistics
- Variational methods
- Likelihood-free/indirect methods
- Symbolic data analysis
- Extreme value theory
Eva Stadler (The Kirby Institute)
- Statistical analysis of data relating to infectious diseases (malaria or COVID-19)
- Developing mathematical methods of topological data analysis
- Applications of topological data analysis for genetic and clinical data
- Analysis of large spatial datasets
- High-dimensional data analysis
- Simulation-based inference
- For more, see UNSW Eco-Stats projects ideas
- Extreme value theory